LLM fine-tuning is an essential process in the realm of machine learning and natural language processing (NLP). It involves taking a pre-trained large language model (LLM) and adapting it to perform specific tasks or generate content that aligns with particular requirements. Understanding LLMs begins with recognizing their foundational architecture, which is built upon vast datasets that enable them to grasp language patterns, context, and semantics.
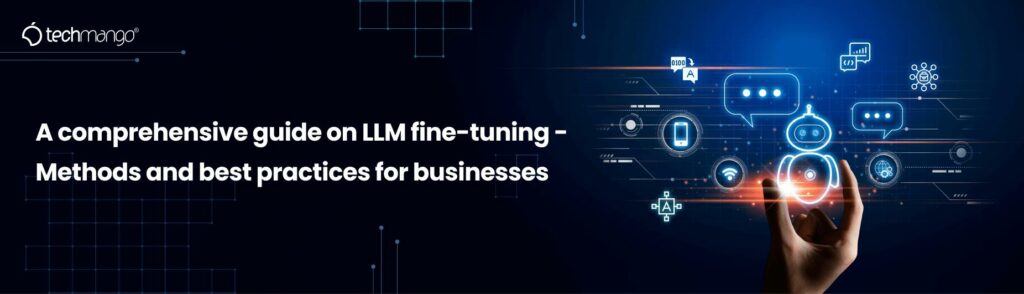
Fine-tuning allows practitioners to leverage the general knowledge encoded in these models while tailoring them for specialized applications. This process typically requires a smaller dataset that reflects the target domain or task, ensuring that the model can produce relevant and accurate outputs. By adjusting parameters and training on this focused dataset, users can enhance the model’s performance for specific use cases—be it sentiment analysis, chatbot responses, or even creative writing.
For those familiar with machine learning basics, fine-tuning represents a middle ground between training a model from scratch and using a generic pre-trained version. It efficiently harnesses existing capabilities while optimizing them for unique challenges within various industries. As organizations increasingly adopt LLMs to improve their operations and customer interactions, understanding LLM fine-tuning will be crucial for maximizing their potential in real-world applications.
In this blog we will see why fine-tuning LLMs is essential for businesses, some best practices in LLM fine-tuning, notable challenges that may arise in the process, and the best place to attain your entrepreneurial goals.
The importance of fine-tuning LLMs for business apps
Fine-tuning LLMs for business applications is crucial in today’s competitive landscape. By customizing these models, organizations can enhance model performance to meet specific needs and objectives. This process involves adjusting the pre-trained LLMs with domain-specific data, allowing them to understand and generate content that aligns closely with a company’s unique requirements.
Tailored AI solutions derived from fine-tuned LLMs give businesses a significant edge over their competitors. For instance, companies can leverage these customized models to improve customer service through more accurate chatbots, generate targeted marketing content that resonates with their audience, or streamline internal processes by automating routine tasks.
Ultimately, the importance of fine-tuning LLMs lies in their ability to transform generic AI capabilities into powerful tools that drive efficiency and innovation within various business applications. As organizations increasingly recognize the value of bespoke AI solutions, those who invest in fine-tuning will likely gain a substantial competitive advantage in their respective industries.
Related Blog
Techmango’s AI-powered Software Development Life Cycle: From Vision to Value
Understanding the different methods of LLM fine-tuning
Understanding the different methods of LLM fine-tuning is crucial for anyone looking to leverage these powerful tools effectively. At the heart of this process lies transfer learning, a technique that allows models pre-trained on vast datasets to be adapted for specific tasks with minimal data and effort. This not only saves time but also harnesses the extensive knowledge embedded in these models.
Supervised fine-tuning takes this a step further by utilizing labeled datasets, enabling models to learn from examples and make predictions based on specific inputs. This method is particularly effective when you have a clear set of objectives and ample data.
On the other hand, unsupervised fine-tuning relies on unlabelled data, allowing models to learn patterns and structures within the language without explicit guidance. This flexibility makes it an attractive option for scenarios where labeled datasets are scarce or unavailable.
Prompt tuning offers another innovative approach by optimizing prompts rather than altering model parameters directly. This method can yield impressive results with minimal changes, showcasing how nuanced adjustments can lead to significant improvements in performance.
Lastly, few-shot learning stands out as a revolutionary technique that enables LLMs to perform tasks with little training data—making it incredibly useful in dynamic environments where rapid adaptation is necessary.
By understanding these diverse methods of fine-tuning, practitioners can select the right approach tailored to their specific needs, thus maximizing the potential of LLMs in their projects.
Case study
LLM-powered app for safer machinery troubleshooting
Best practices for successful LLM fine-tuning in your organization
Fine-tuning LLMs within your organization can unlock unprecedented capabilities, but success hinges on adhering to best practices that ensure optimal performance.
First and foremost, data preparation strategies are paramount. Start by choosing the right datasets that align with your specific objectives—consider both the quality and diversity of the data. This not only ensures relevance but also enhances model generalization.
Next, dive into hyperparameter tuning tips. This is where you can significantly influence model performance. Experiment with different learning rates, batch sizes, and other parameters systematically to identify what yields the best results for your unique use case.
Finally, establish robust evaluation metrics for fine-tuned models. These metrics will not only help gauge how well your model performs but also inform further adjustments in training or data selection. Metrics like accuracy, F1 score, or perplexity should be tailored to reflect the specific goals of your project.
By adhering to these best practices—data preparation strategies, careful dataset selection, hyperparameter tuning tips, and effective evaluation metrics—you set a solid foundation for successful LLM fine-tuning in your organization that drives tangible outcomes and innovation.
Common challenges and how to overcome them in LLM fine-tuning
LLM fine-tuning can present several common challenges that, if not addressed, may hinder the effectiveness of your model. To overcome certain basic hurdles, it’s crucial to curate high-quality datasets that reflect the diverse contexts and nuances your model will encounter in real-world applications.
Another significant hurdle is overfitting, where the model performs well on training data but poorly on unseen data. To counteract this, implement techniques such as regularization and cross-validation during the fine-tuning process. These methods help ensure that your model generalizes well beyond its training examples.
Additionally, resource management is a vital consideration; fine-tuning LLMs often requires substantial computational power and time. To mitigate this challenge, leverage cloud-based solutions or optimized frameworks that enable efficient training processes without exhausting resources.
Employ continuous evaluation strategies to identify drift in accuracy over time and adapt your fine-tuning approach as necessary.
By addressing these common challenges head-on with strategic solutions, you can enhance the performance and reliability of your fine-tuned language models, ensuring they deliver meaningful results in their intended applications.
The future of LLM fine-tuning: Trends and opportunities for businesses
The future of LLM fine-tuning is brimming with exciting advancements that businesses cannot afford to overlook. As we stand on the brink of a new era in artificial intelligence, understanding these upcoming advancements is crucial for shaping effective corporate AI strategies.
One of the most promising areas lies in customer service automation. Imagine a world where AI can handle complex customer inquiries seamlessly, providing personalized responses and resolving issues without human intervention. This isn’t just a distant dream; it’s an imminent reality as LLMs evolve to understand context and nuances better than ever before.
Furthermore, companies must stay attuned to future trends that will inevitably impact their AI strategies. Organizations leveraging fine-tuned models can expect heightened efficiency, cost savings, and enhanced customer satisfaction. The opportunities are vast—ranging from improved data analysis capabilities to more sophisticated content generation.
By investing in LLM fine-tuning now, businesses position themselves at the forefront of innovation, ensuring they harness these powerful tools effectively to meet their evolving needs and outpace competitors in this rapidly changing landscape.
Conclusion: Consult Techmango and leverage the power of LLM fine-tuning for your business growth!
From the above sections, you will be now clear that the opportunity to leverage LLM fine-tuning for your business growth is not just a trend—it’s a necessity. And for that, it becomes a must to make use of the best-in-class LLM fine-tuning services. Here is where Techmango’s advanced fine-tuning processes come into the picture.
Techmango is a renowned IT service provider offering impeccable IT services and solutions for multitudinous business models worldwide. It also excels in providing GenAI, AI/ML services that will enable you to swiftly attain your business goals, resulting in long-term sustainable growth.
So, with our team of adept professionals, customizing the power of LLMs to your data with advanced fine-tuning processes will be seamless. Make it more accurate to your domain-specific details and secure the sensitive data within the organization.
Now is the time to take action. Invest in understanding how LLM fine-tuning can be integrated into your strategies. Collaborate with our experts and explore user-friendly platforms that make this technology accessible. Seize this moment to elevate your business and watch as it thrives in ways you never thought possible!